Predictive Analysis to foster a more
Evolved Experience for the User
What is Predictive Analysis
The technology used in predictive analysis, in the form of machine learning algorithms, statistics and data-mining techniques, helps Like Reply in the development of models and emerging trends in the data gathered.
Using predictive analysis, the Like Reply team develops a positioning strategy for the company that offers the advantage of intuition and the interpretation of online and offline phenomena.
Multichannel and centralized customer focuses to forecast latent needs
In the current competitive environment, the different multichannel and centralized focuses among customers call for new ways of utilizing data to anticipate customers’ latent needs.
Organizations that have tools available for reading and interpreting data are supported in the management of difficult decisions, with greater efficacy in results: in attracting customers, in retaining them, and in maximizing profits.
Our Goals
Recommendation Systems
Recommendation systems are developed with the aim of predicting customers’ interests (what they want to buy or look at) and providing them with specific suggestions. This takes place by means of statistics and machine learning algorithms, with the intention being to maximize profits. When a customer purchases a product or shows an interest in it, the system automatically recommends a product or service that it considers relevant for that customer. Recommendation systems are based, for example, on the concepts of collaborative filtering or content-based filtering, i.e. the possibility of making suggestions through the identification of similar users based on the interests shown.
Target of Marketing Campaigns
Predictive analysis molds digital marketing campaigns so they are aimed at the customer, narrowing down the advertisements to high-potential segments specific to the user. Like Reply can attribute – either to a prospective customer or to a customer who has already been acquired – a category denoting the probability of conversion based on multiple factors that are analyzed by the predictive algorithm (duration of session, number of pages visited, source of traffic, stage of advancement through the funnel, choice of promo, CRM info, etc.). By creating an effective predictive model for the classification of customers based on the greatest possibility of conversion, the team can export these segments towards the tools delivering ad campaigns and thus increase the return on investment, profitability, the conversion rate and customer satisfaction.
Attribution to Online and Offline Marketing Channels
Attribution analysis with respect to profits and conversion via media channels reaches its limits in traditional, determinist models (in particular that of “last click”). These models very rarely offer the option of analyzing the effective contribution, since users’ conversion paths tend to involve several stages and comprise different phases of advancement through the funnel. Use of these models can sometimes result in a short-sighted approach that tends to exclude moments of conversion in which media channels frequently play a determined rather than considered role. Furthermore, such models do not take into account the multi-device experience and the impact of traditional marketing channels (TV, press, radio, etc.) integrated with the digital ones. Hence, Like Reply offers a structured approach in the transition from a determinist model to a predictive model, thus optimizing the media budget and maximizing profits. The attribution methodology is based on the application of sophisticated algorithms to the datasets collected, assigning a partial conversion credit to the marketing touchpoints that effectively contribute to conversion, irrespective of their position within the funnel.
Customer Lifetime Value
The customer lifetime value (LTV) is an indicator based on a predictive model that shows how worthwhile it is maintaining a relationship with a specific customer over time. It indicates the value of the future profit that may be generated if the relationship with the customer is maintained. It is an important metric that is hard to calculate, since it takes into account different variables, including the cost of customer acquisition (CAC), the retention rate, the churn rate, repeated purchases, etc. The customer lifetime value makes it possible to monitor the course of business and to take relevant strategic decisions; indeed, it is often more advantageous for a company to focus its own marketing efforts on customer segments that it has already acquired, which may be profitable in the long term, rather than acquiring new customers who only make it possible for the company to achieve short- or medium-term goals.
Alert Systems
Personalization and Optimization of the Customer Journey
After the company has collected sufficient data on the actions taken by its own customers, predictive analysis makes it possible to identify similar patterns in user behavior and thus facilitates personalization of the experience, be it in terms of the content offered or modifications to the digital interface displayed to the user.
Predictive analysis adds another layer to the techniques used in CRO, the experimental research methodology that facilitates optimization of the conversion rate of digital applications. By means of predictive analysis, Like Reply evaluates all the paths taken by users on a digital platform, identifying the content that users visit most often before effecting a specific conversion (e.g. registration, lead, purchase). This kind of analysis would be impossible using descriptive analysis tools, since the possibilities, sequences and modes through which the user may interact with a platform can be manifold and very hard to predict in advance.
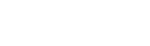
Let’s transform your digital landscape together
Unlock the full potential of your digital presence with our expert marketing strategies designed to drive growth, engagement, and conversion. Connect with us today to transform your vision into success.